In part 1 of this blog, we presented artificial intelligence (AI) as a solution that can help law enforcement analyze large data sets, recognize trends, and pinpoint shooters before they have a chance to carry out an attack.
Although AI has been a field of study for decades, applying it to law enforcement can be a difficult process. The challenges of detecting a potential mass shooter include:
- Too many data points
- Combining data stored in different formats across various databases
- Recognizing data that appears harmless in isolation, but raises a red flag when combined with other data
For AI to succeed in this regard, it needs to be capable of traversing multiple data sets, understanding the relationships between them, and recognizing potential threats while minimizing the rate of false positives.
Building a Risk Model for Mass Shooters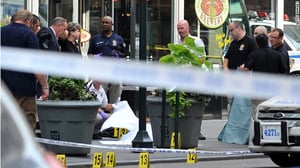
Examining all available data points related to previous mass shooters reveals numerous indicators on which we can begin to construct a common risk model. If we look at school shootings in particular, we see that most incidents involved current or former students and that these students had several ‘red flags’ that were retroactively identified in school, law enforcement, medical, or public records. Fusion Centers already collect much of this information for the convenience of law enforcement, but are not always able to connect these disparate pieces of information in time.
Aggregate data also presents another problem: scale. In order to find individuals at risk, we need to start with enormous data sets and work our way down. For example, imagine we want to generate a list of individuals who have access to firearms, have a history of mental health concerns, and have a criminal record. On their own, none of these factors is particularly troubling but taken together, their risk of becoming a threat increases.
Consider these numbers:
- Around 85 million Americans have a criminal record
- Around 40 million Americans are currently taking psychoactive drugs
- Around 135 million Americans live in a house with a firearm
The risk of an individual becoming a mass shooter– even if still low – exponentially increases for an individual who fits into all three of these categories. Adding additional criteria to the profile changes the scenario significantly:
- Has a record of violence against other students including suspensions or expulsions
- Is associated with online hate groups
- Has a history of sending threatening messages over social media
These factors are increasingly more damaging and when taken together, paint a much more sinister motive. Clearly the more conditions we introduce, the easier it is to identify high-risk individuals. Dr. Geoffrey Barnes explains how,
"[Data stored in criminal records] are combined in thousands of different ways before a final forecasted conclusion is reached. Imagine a human holding this number of variables in their head, and making all of these connections before making a decision. Our minds simply can't do it."
The problem is that each of these data points resides on separate platforms. School records, protected medical records, and social media databases are all disconnected from one another. To identify threats effectively, law enforcement needs to fuse these disparate data sources together, and then apply AI to meaningful insights.
Example: A Threatening Facebook Post
Consider a student who posts a threatening message saying "I feel like killing someone today" on Facebook. Posts like these are nothing new: 66% of U.S. adults have witnessed harassing behavior online, and 18% have experienced particularly severe forms of online harassment. Without context, it's impossible to know if posts like these are just harmful posts or if they present a greater threat.
Now assume that after searching local gun stores, we find out that the student purchased a handgun just a few days before writing the post. We also find by looking at his school records that the student got into a fight and was suspended as a result. Now the post takes on an entirely new meaning, and the risk of violence becomes that much greater raising many red flags to LEAs.
The Challenge of “Too Much Data”
Collecting data in larger databases helps us derive more concrete conclusions, but only if we have a way of analyzing it all together. If analysts had to sift through this information manually, the sheer volume of information would be overwhelming. It would be impossible to generate meaningful or actionable conclusions in a timely manner.
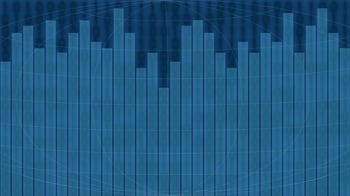
This is where AI excels. AI-powered systems scan through disparate data sets far more quickly and effectively than human analysts can, automatically identifying individuals that match or exceed specific, defined risk parameters. For example, a system can be configured to assess the threat level for individuals meeting certain criteria including age, previous arrest record, investigations, complaints, and any previous records of school violence, threats, bullying, and/or indictments.
As AI becomes more sophisticated, the insights it generates becomes more immediately useful and actionable to analysts. Ultimately, AI can enable a mechanism for preventing, or reducing, the number of mass shootings.
Conclusion
Law enforcement often has the data at their disposal, but need the right tools to analyze it. With data fusion and AI, law enforcement has a much greater chance of identifying potential shooters before they have a chance to attack. With the prevalence of mass shootings across the United States, the importance of aggregating data and using tools to identify individuals who are at high risk for conducting mass shootings is more important than ever.